Machine Learning-Powered Natural Terrain Landslide Identification and Susceptibility Assessment
Doctoral Thesis Research
Wang, H. (2020). Machine learning powered natural terrain landslide identification and susceptibility assessment. Ph.D. Thesis, The Hong Kong University of Science and Technology. https://doi.org/10.14711/thesis-991012880263503412
Abstract
In recent years, extreme weather has brought about many disasters worldwide, including devastating natural terrain landslides, causing significant casualties and economic loss. To effectively mitigate natural terrain landslides risk, landslide identification and susceptibility assessment become increasingly important, especially for cities like Hong Kong whose urban area and population are largely located on or close to steep hillsides. Meanwhile, in the big data era, everchanging machine learning techniques enable new opportunities for both landslide identification and susceptibility assessment. Hence, the principal objective of this thesis research is to use state-of-art machine learning techniques to develop a series of machine learning powered methods to improve and advance landslide identification and susceptibility assessment and to contribute to the fight against natural terrain landslide hazard in Hong Kong and other parts of the world in the 21st century.
To begin with, a new database, the Rainstorm and natural tErrain lAndslide Database of Hong Kong (READHK), is first established on the basis of six data sources of Hong Kong (i.e., historical rainstorm records, natural terrain landslide inventory, high resolution digital terrain model, hourly historical rainfall data, geological maps and multi-spectral remotely-sensed images), providing comprehensive data support for analysing historical rainstorms and natural terrain landslides in Hong Kong. Then, an integrated landslide location identification method which is able to identify both relict and recent landslides from digital terrain model using machine learning and deep learning is proposed and validated on Lantau Island, Hong Kong. In addition, a multi-scale landslide identification method using transfer learning is developed to enhance the landslide location identification in areas with insufficient landslide records. Apart from location identification, an integrated machine learning powered automated landslide boundary identification method is also developed for co-seismic landslides, serving as the very first work which automatically produces high-resolution (pixel-level) and high-accuracy detection results for co-seismic landslides and applies backscatter data to automated landslide detection.
After that, a novel AI and object-based landslide susceptibility assessment method is proposed and validated in Hong Kong. For the first time, the hybrid network architecture of convolutional neural network and long short-term memory (CNN-LSTM) and the bidirectional long short-term memory architecture of recurrent neural network (BiLSTM-RNN) are successfully applied to landslide susceptibility analysis. It is also the first time to assess landslide susceptibility for the entire Hong Kong using AI techniques. In addition, a transfer learning based efficient landslide susceptibility assessment method is developed and validated. In order to conduct dynamic landslide susceptibility assessment using slope performance information, novel physically-based methods for updating landslide susceptibility using Bayesian approaches are proposed and validated with case studies, which are able to consider the inherent correlations in landslide susceptibility analysis, as well as update regional landslide susceptibility and soil parameters according to slope performance information, effectively improving the accuracy and capacity of physically-based landslide susceptibility analysis.
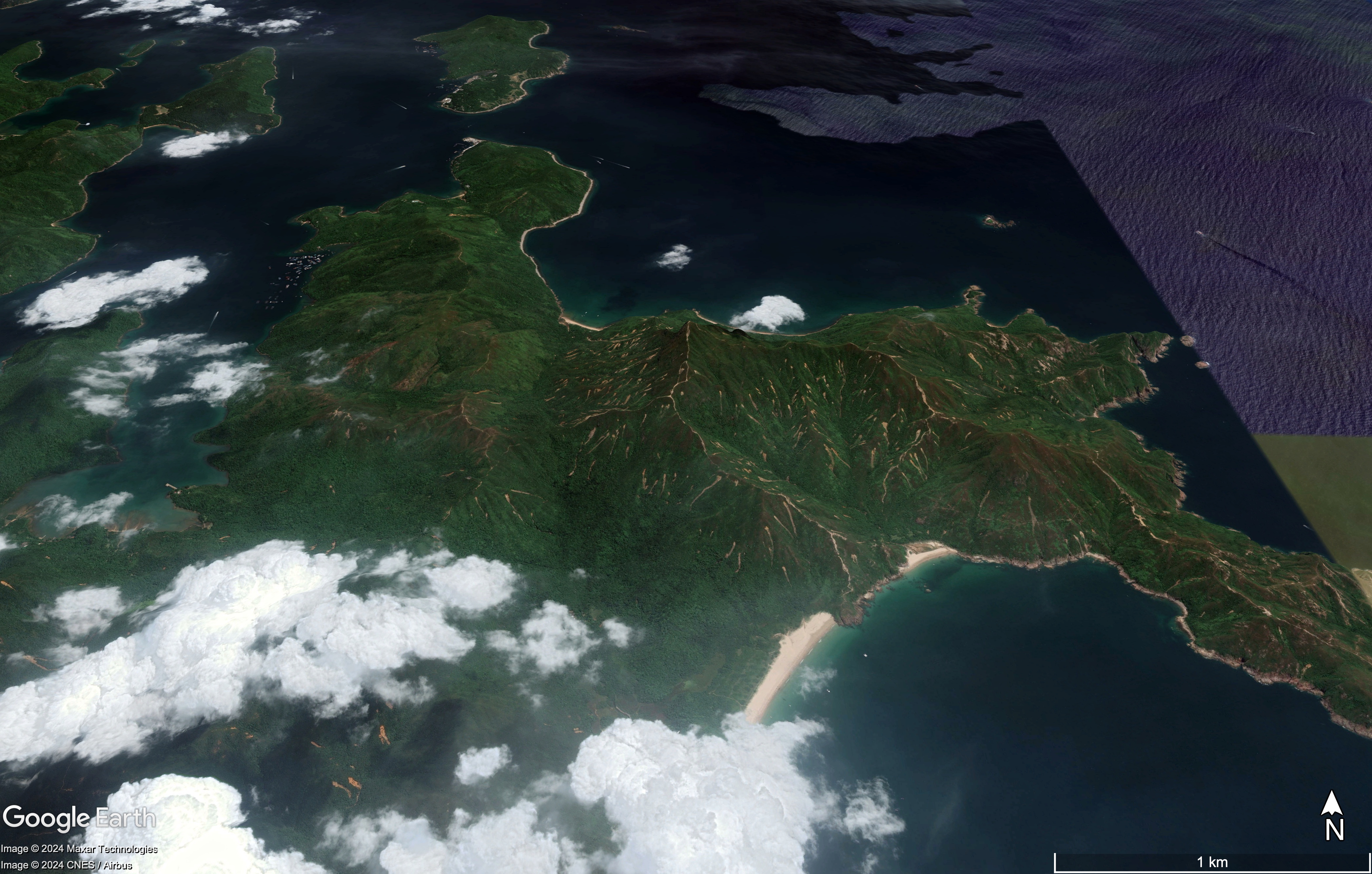
Natural terrain landslides in Hong Kong